Bias in Healthcare AI: How Open-Source Collaboration Can Build Fairer Algorithms for Better Patient Care
Table of Content
The integration of artificial intelligence (AI), particularly large language models (LLMs) and machine learning algorithms, into healthcare has transformed the industry dramatically. These technologies enhance various aspects of patient care, from diagnostics and treatment recommendations to continuous patient monitoring.
However, the application of AI in healthcare is not without challenges. One significant concern is the inherent bias within AI algorithms, which can lead to serious consequences in patient outcomes.
This article delves into how open-source collaboration can help mitigate these biases, promoting a more equitable and effective healthcare system.
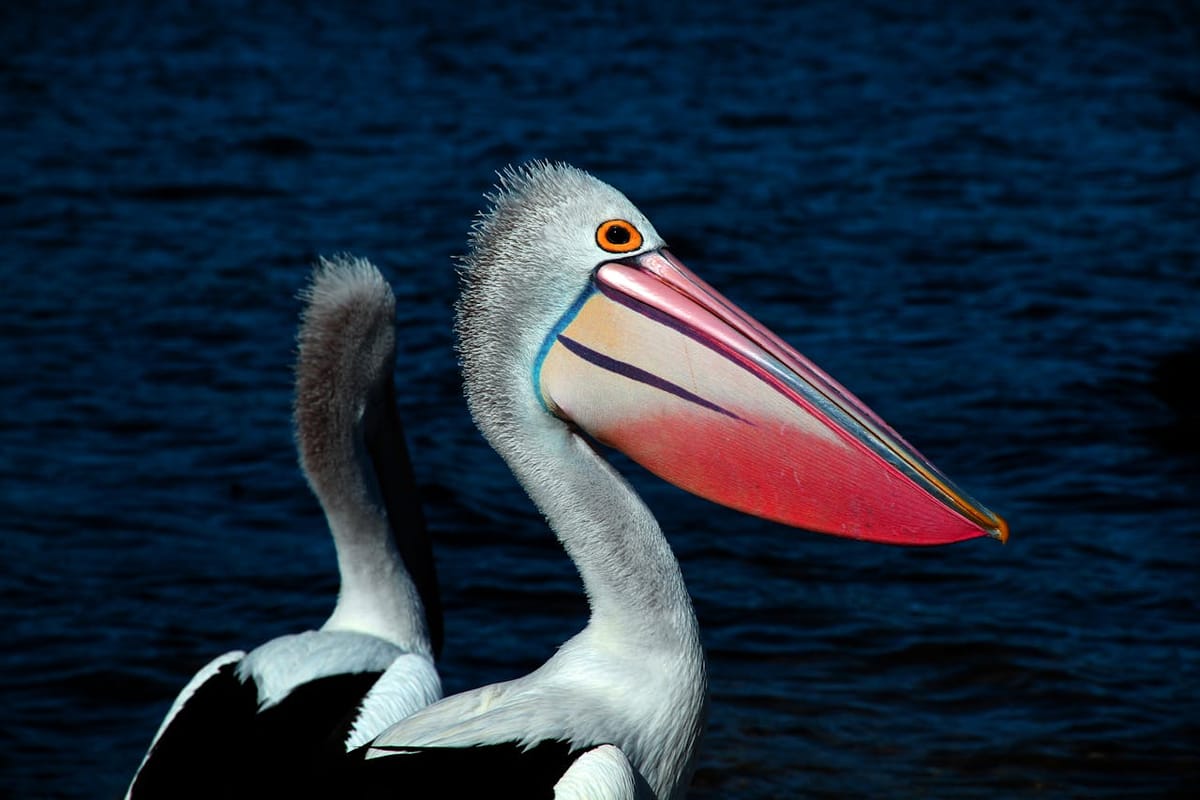
How Bias Creeps into Healthcare AI Algorithms
1- Data Bias
Often, AI systems are only as good as the data they're trained on. A common pitfall is the underrepresentation of certain demographics in training datasets, leading to algorithms that perform poorly for women or people of color. For instance, an algorithm trained predominantly on data from white males might fail to accurately diagnose conditions prevalent in other ethnic groups.
2- Algorithmic Bias
The choices made during the design and development of AI systems can inadvertently introduce bias. This includes the selection of features to be used by the algorithm and the optimization goals set during its training.
3- Human Bias
The unconscious biases of developers can also seep into the algorithms. The assumptions and preferences of those who code and design AI systems can influence the final outcomes, often at the expense of objectivity.
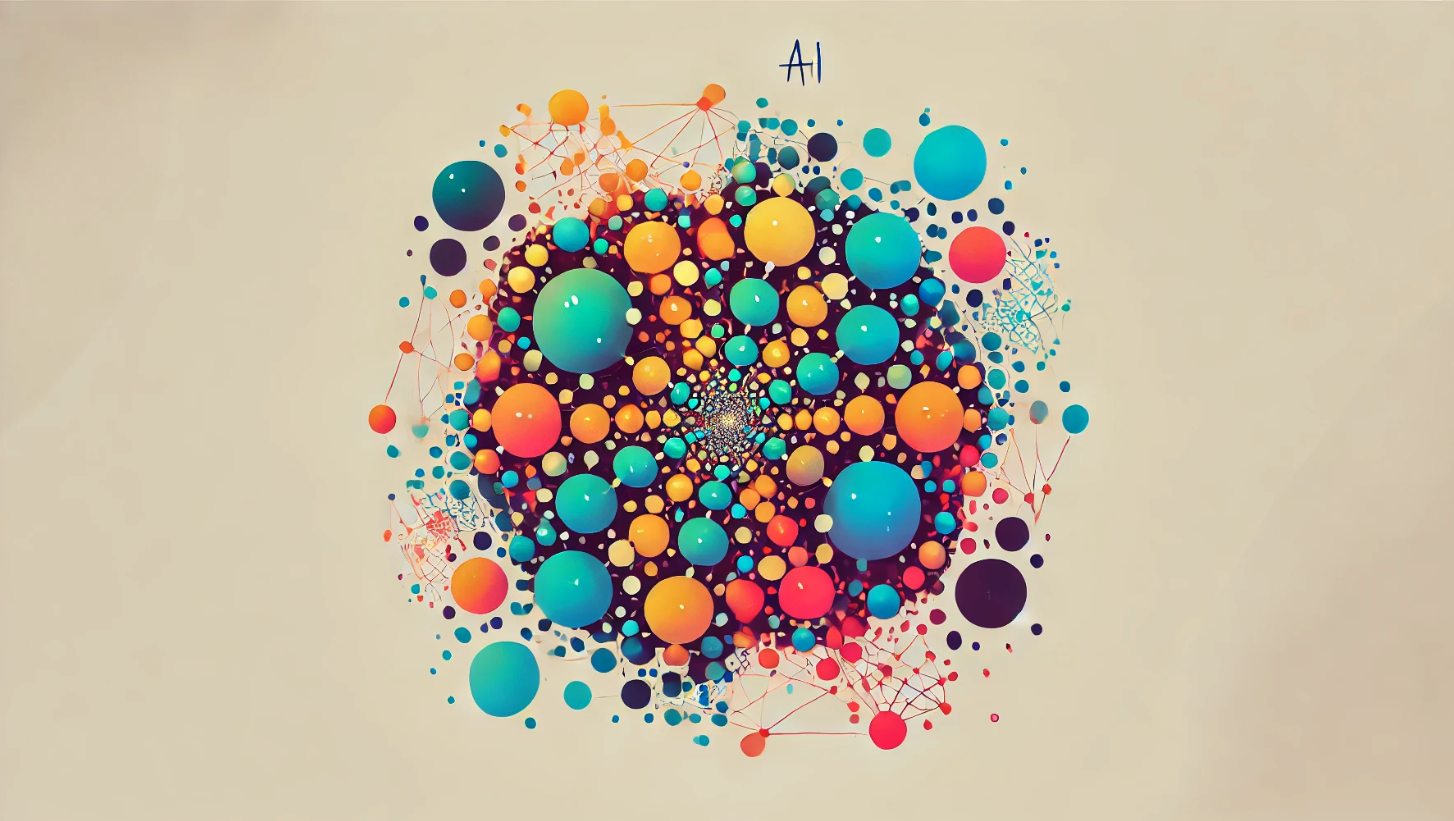
The Impact of Bias on Patient Care
Misdiagnosis and Inequitable Treatment:
There are alarming case studies of AI systems that have failed to diagnose conditions accurately in underrepresented groups. Such inaccuracies can lead to misdiagnosis or inappropriate treatment recommendations.
Exacerbating Health Disparities:
When AI algorithms are biased, they can worsen existing disparities in healthcare access and outcomes, particularly for marginalized communities.
Loss of Trust in AI Systems:
Biased algorithms can erode trust in AI technologies, both from healthcare providers and patients, which is detrimental to the broader adoption of these potentially life-saving systems.
Open-Source Collaboration: A Path to Fairer AI
Transparency and Accountability
Open-source projects inherently promote a level of transparency not always present in proprietary systems. By making the source code available, these projects allow for greater scrutiny and accountability of the algorithms and their underlying data.
Diverse Contributions
Open-source projects benefit from contributions by a global, multidisciplinary community. This diversity helps in recognizing and correcting biases that might not be evident to a more homogenous group.
Community-Driven Improvements
There are examples of open-source healthcare AI projects where community feedback has led to significant improvements in reducing bias. This collective input is crucial for developing more equitable AI systems.
Strategies for Building Fairer AI Algorithms
Diverse and Representative Datasets:
It’s crucial to ensure that AI systems are trained on diverse and representative datasets. This approach helps in creating algorithms that perform well across various demographics.
Bias Detection Tools:
Several open-source tools are available that help in identifying and mitigating bias in AI algorithms. These tools are essential for developers looking to build fairer systems.
Ethical AI Frameworks:
The adoption of standardized guidelines and best practices in AI development is vital. These frameworks help ensure that AI systems are ethically developed and deployed.
Continuous Monitoring and Feedback Loops:
AI systems must be continuously monitored and updated post-deployment to ensure they remain effective and fair over time.
Challenges and Future Directions
Barriers to Open-Source Adoption:
Challenges such as funding, intellectual property issues, and a lack of clear incentives can hinder the adoption of open-source practices.
The Role of Policy and Regulation:
Policymakers and institutions have a crucial role in supporting the development of open-source AI, ensuring it can be a viable solution for future healthcare needs.
The Future of Fair AI:
Looking forward, the role of open-source collaborations in shaping fairer AI systems in healthcare is optimistic yet requires concerted effort across various sectors.
Conclusion: Building a Fairer Future for Healthcare AI
Addressing bias in AI algorithms is crucial for the advancement of healthcare technologies. Through open-source collaboration, we can steer the development of AI towards more equitable outcomes. By leveraging the collective expertise of a global community, ensuring continuous feedback, and upholding high ethical standards, we can ensure that AI systems benefit all segments of society equally.
This approach not only enhances the efficacy of patient care but also fosters a broader acceptance and trust in AI applications within healthcare.