AI Terminology for Healthcare Professionals: A Simple Guide
Artificial Intelligence (AI) is revolutionizing healthcare, introducing innovative tools for improved diagnostics, patient care, and medical research. As AI becomes increasingly prevalent in healthcare, it's crucial for doctors and medical professionals to grasp key AI terminology.
In this guide, we'll explain essential AI concepts in simple terms and highlight their significance for healthcare professionals.
Why Doctors Should Care About These AI Terms
AI is already transforming healthcare through advanced diagnostics, personalized treatments, predictive analytics, and robotic surgery.
As AI integrates further into clinical workflows, doctors must grasp how these technologies function to make informed decisions about their use.
Understanding AI terminology enables medical professionals to interact effectively with AI systems, ensuring they leverage the right tools to enhance patient care.

Key AI Terms Every Doctor Should Know
1. Machine Learning (ML)
What it is: Machine learning is a subset of AI where computers learn from data and improve their performance over time without explicit programming. It uses algorithms to recognize patterns and make predictions or decisions based on data.
Why it matters: In healthcare, ML is crucial for medical diagnostics, predicting patient outcomes, and analyzing medical images. Doctors leverage machine learning to identify complex patterns in medical data that humans might miss or take longer to detect.
2. Large Language Models (LLMs)
What it is: Large Language Models (LLMs) are AI systems trained on extensive text data to comprehend and produce human-like language. Notable examples include GPT-4 and Gemini.
Why it matters: In healthcare, LLMs power applications like automated documentation, patient-interaction chatbots, and clinical study summarization. These models enable doctors to efficiently process and condense vast amounts of medical literature.
3. Deep Learning
What it is: Deep learning is an advanced form of machine learning that employs multi-layered neural networks to analyze vast amounts of data. It excels at processing complex information like images, speech, and intricate datasets.
Why it matters: In healthcare, deep learning models play a crucial role in medical imaging—detecting tumors in MRI or CT scans and analyzing pathological slides. This technology has transformed radiology and pathology by significantly improving diagnostic accuracy.
4. Prompt Engineering
What it is: Prompt engineering is the art of crafting and refining input commands (prompts) for AI systems like Large Language Models to produce accurate and relevant outputs.
Why it matters: As AI integrates into clinical workflows, prompt engineering ensures that AI tools deliver meaningful and reliable responses. This is particularly important when automating tasks such as generating clinical notes or answering patient queries. By mastering prompt engineering, doctors can harness AI's full potential in medical decision-making.
5. Natural Language Processing (NLP)
What it is: Natural Language Processing (NLP) enables computers to understand, interpret, and respond to human language in a meaningful way.
Why it matters: NLP is crucial in healthcare for medical transcription, processing clinical notes, extracting relevant data from unstructured text, and powering patient-interactive virtual assistants. By automating paperwork, NLP allows doctors to dedicate more time to patient care.
6. Artificial Neural Networks (ANNs)
What it is: ANNs are algorithms inspired by the human brain's structure. They consist of interconnected layers of nodes (neurons) that process and analyze complex datasets.
Why it matters: In healthcare, ANNs excel at diagnosing diseases from patient data, predicting outcomes, and analyzing medical images. Doctors using AI-based diagnostic tools benefit from understanding how ANNs inform decision-making processes.
7. Reinforcement Learning
What it is: A type of machine learning where an AI system learns through interaction with its environment, receiving feedback as rewards or penalties.
Why it matters: In healthcare, reinforcement learning optimizes personalized treatment plans by learning from patient responses. It also enhances robotic surgery, allowing systems to improve precision based on previous outcomes.
8. Robotic Process Automation (RPA)
What it is: RPA employs software "robots" to automate repetitive tasks—such as data entry or appointment scheduling—without the need for complex programming.
Why it matters: By automating administrative tasks in healthcare, RPA reduces errors and frees up staff to focus on patient care. This allows doctors to dedicate more time to clinical tasks while routine operations are efficiently handled in the background.
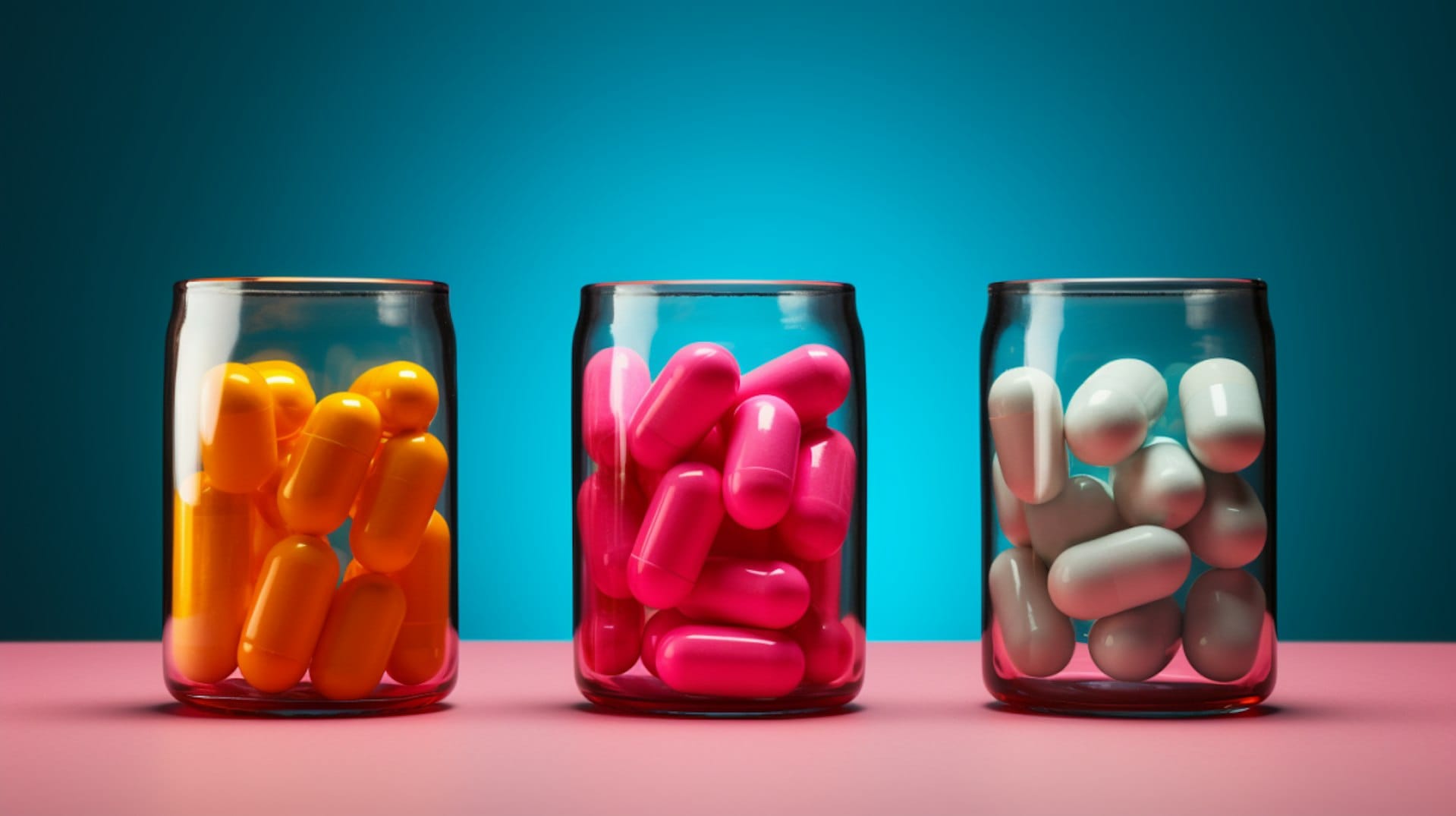
How to Create and Write Prompts for AI
Prompt engineering is crucial when working with AI systems like LLMs. Here's a concise guide to crafting effective AI prompts:
- Be Specific: Clearly define the task. AI models thrive on detailed instructions.
- Example: "Summarize the patient's history, focusing on respiratory issues."
- Provide Context: Include relevant background information when necessary.
- Example: "Using the patient's previous lung function test results, recommend a treatment plan."
- Use Simple Language: Avoid jargon unless the AI is trained in domain-specific vocabulary.
- Example: "Explain the proposed treatment's benefits in patient-friendly terms."
- Guide the Output: Specify the desired format or structure for the response.
- Example: "List the disease symptoms in bullet points."
- Experiment and Refine: Don't hesitate to adjust prompts. Small tweaks can significantly improve accuracy.
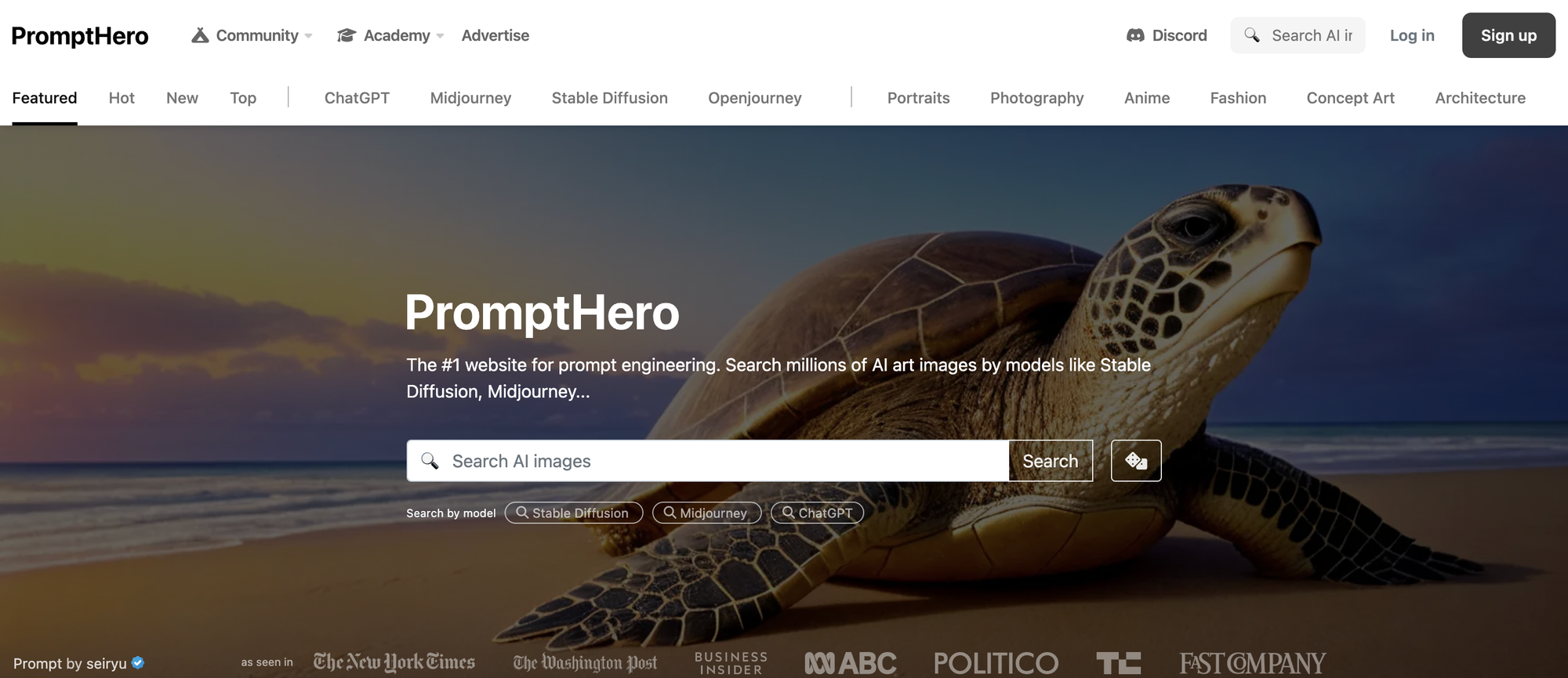
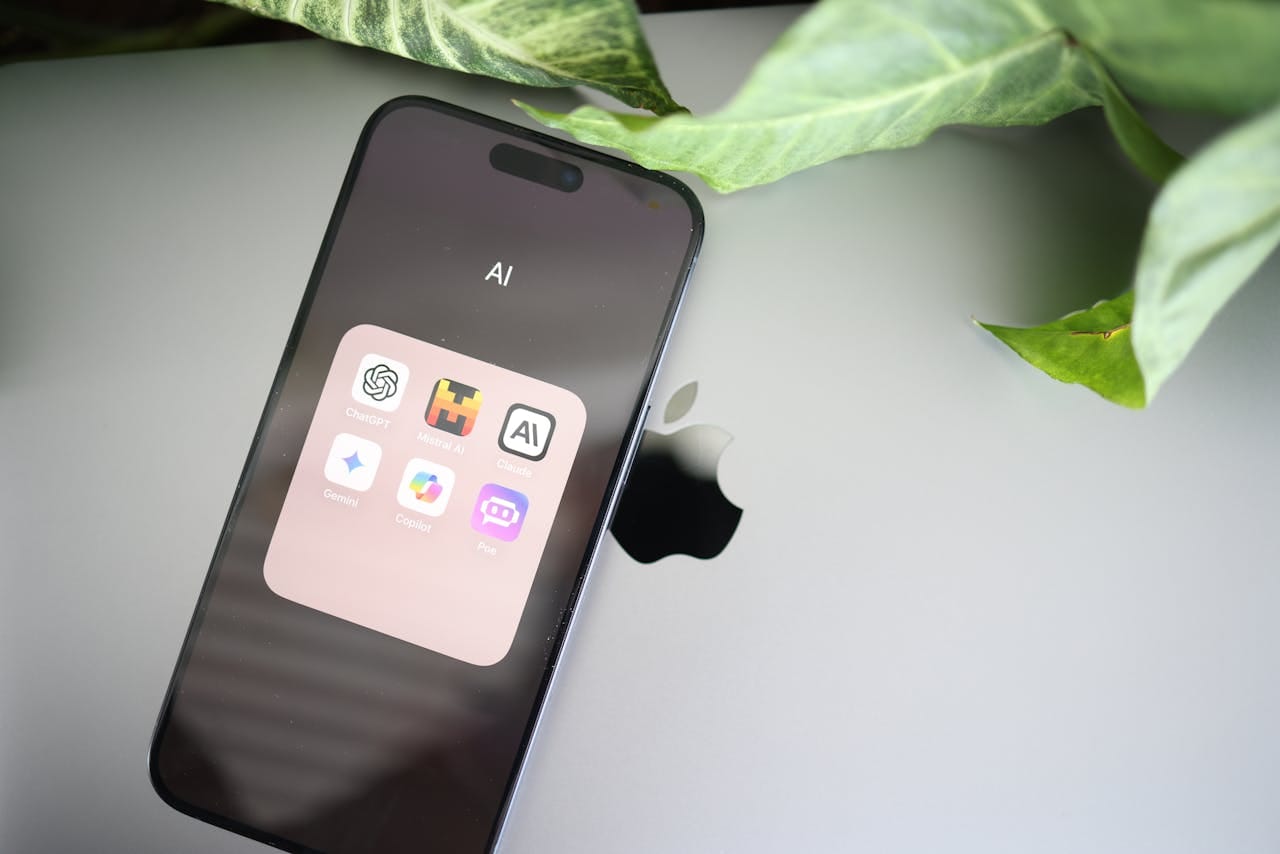
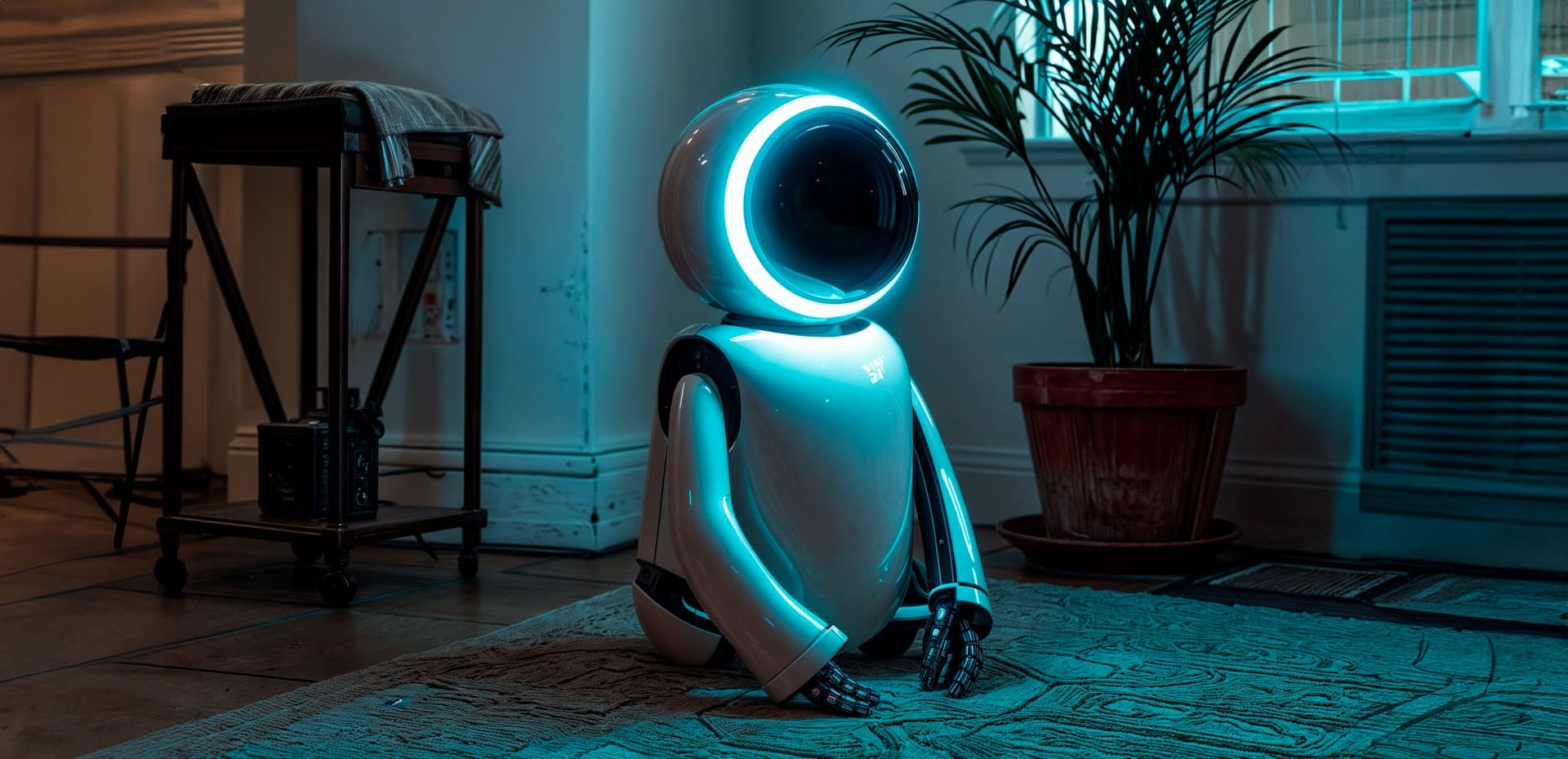
FAQs on AI for Medical Professionals
1. Why should doctors learn about AI?
AI is transforming healthcare, from diagnostics to treatment planning. Understanding AI enables doctors to use these tools effectively, enhancing patient care and improving decision-making.
2. What's AI's role in diagnostics?
AI, particularly machine learning and deep learning, can analyze medical images, lab results, and patient data faster—and sometimes more accurately—than human physicians. This capability aids in early disease detection.
3. How does prompt engineering impact healthcare AI?
Prompt engineering ensures AI systems provide useful, accurate responses. It's crucial when using AI tools to generate clinical notes, patient reports, or summaries.
4. Is AI replacing doctors?
No, AI isn't replacing doctors. Rather, it's a tool that supports doctors by automating repetitive tasks, enhancing diagnostic accuracy, and assisting with data analysis.
5. How can AI improve patient outcomes?
AI can identify trends in patient data, potentially leading to better treatment plans, more accurate diagnoses, and personalized medicine—all contributing to improved patient outcomes.
By grasping these AI concepts and learning to work effectively with AI, doctors and healthcare professionals can enhance patient care, streamline workflows, and remain at the cutting edge of medical technological advancements.